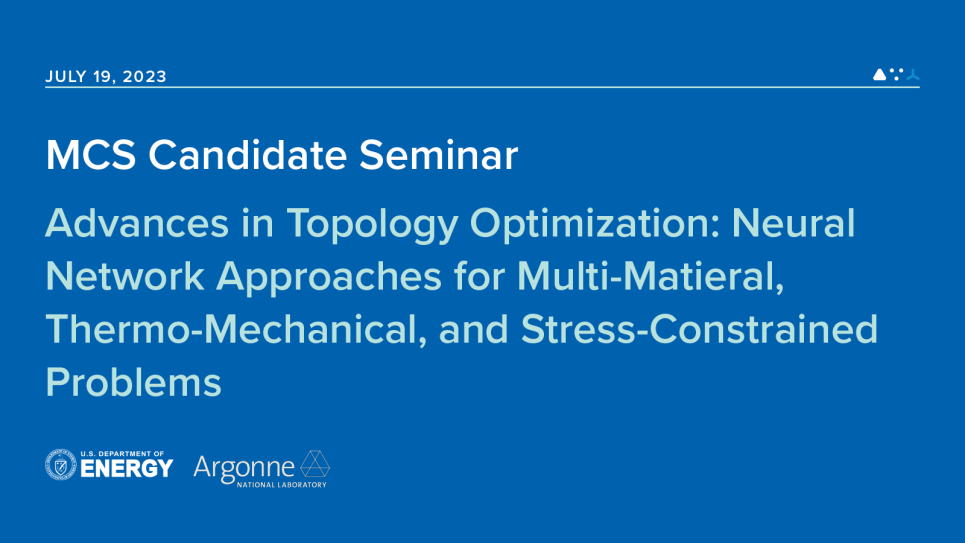
Description:
Lightweight designs are highly desired in aerospace, automotive, and transportation industries for improved structural performance. Topology Optimization (TO) achieves lightweight designs by generating optimal material layouts. However, traditional TO focusing on compliance minimization can lead to high-stress concentrations and failure-prone geometries. Incorporating multi-material, thermos-mechanical, stress-related objectives or constraints is crucial for producing safe and efficient designs. This enables real-time optimization, with the neural network representing the material layout. The network is trained using the JAX library for end-to-end differentiability and automated sensitivity computations. A Fourier space projection controls the length scale of design features, meeting manufacturing requirements. This approach accommodates multi-material, thermo-mechanical, and stress-related objectives, or constraints, ensuring safe and efficient designs. By eliminating explicit sensitivity calculations and enabling real-time optimization, this methodology enhances efficiency and accuracy in achieving lightweight designs.