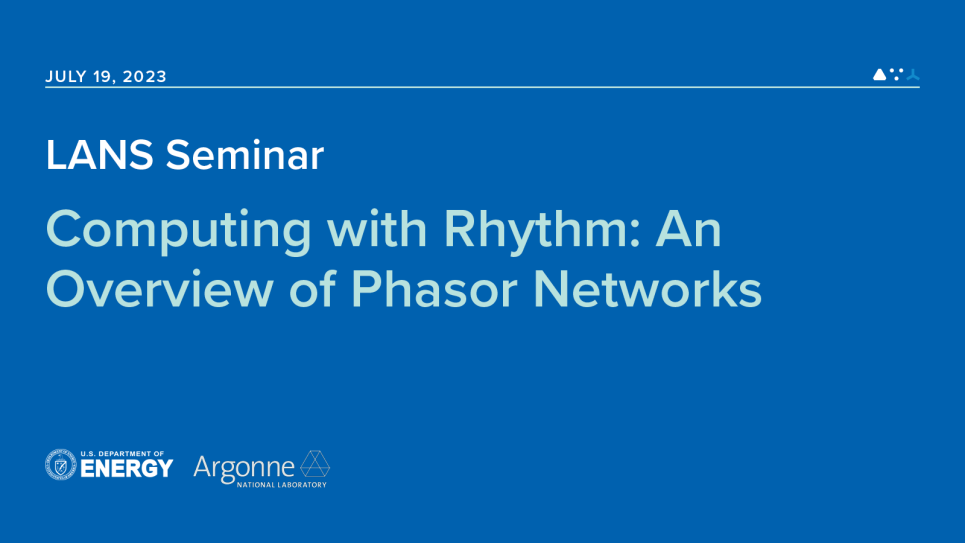
The explosively growing computational needs of modern AI models combined with the slowdown of Moore’s Law creates a multitude of issues, from a lack of reproducibility and open access to large carbon footprints. One path to alleviate these issues seeks to improve computational efficiency for AI by creating systems which utilize biological principles of computation discovered in the brain. ‘Phasor networks’ are one such system, in which activations in a neural network represent the relative phase of oscillators. I present an overview of this interpretation of neural networks and the advantages which it can bring, including the ability to manipulate neural layers algebraically and links to temporal, analog computation.
Bio: Dr. Wilkie Olin-Ammentorp is a post-doctoral researcher in the Mathematics & Computer Science Division of the Argonne National Laboratory. He completed his PhD in Nanoengineering at the State University of New York Polytechnic Institute in 2019 and went on to a first post-doctoral appointment at the University of California, San Diego in the Department of Medicine before joining Argonne in 2022. His research focuses on designing, simulating, and benchmarking novel computing architectures which combine the strengths of modern artificial intelligence as well as principles from biological computation.
See all upcoming talks at https://www.anl.gov/mcs/lans-seminars