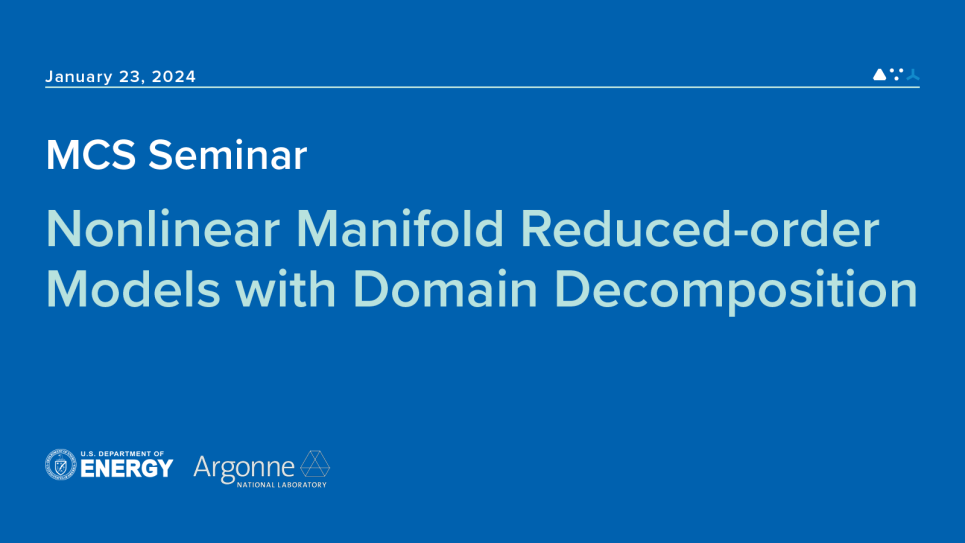
This presentation focuses on integrating nonlinear manifold reduced-order models (NM-ROMs) with domain decomposition (DD). NM-ROMs nonlinearly approximate the full-order model (FOM) state using autoencoders trained on FOM simulation data. NM-ROMs can be advantageous over linear-subspace ROMs (LS-ROMs) for advection-dominated problems, which are difficult to approximate accurately using low-dimensional linear approximations. However, the number of NM-ROM autoencoder parameters requiring training scales with the FOM size, making training costly. To alleviate this cost, DD is applied to the FOM, and NM-ROMs are computed on each subdomain and coupled to obtain a global NM-ROM. Correspondingly, smaller FOM-dimensional training data and significantly fewer autoencoder parameters are required per subdomain NM-ROM than global NM-ROMs. Moreover, sparse autoencoder architectures allow one to apply hyper-reduction, further reducing computational complexity and yielding computational speedup. The proposed DD NM-ROM is compared to a DD LS-ROM on the 2D steady-state Burgers' equation and shows an order of magnitude accuracy improvement.
To add to calendar:
Click on: https://wordpress.cels.anl.gov/cels-seminars/
Enter your credentials.
Search for your seminar
Click “Add to calendar”