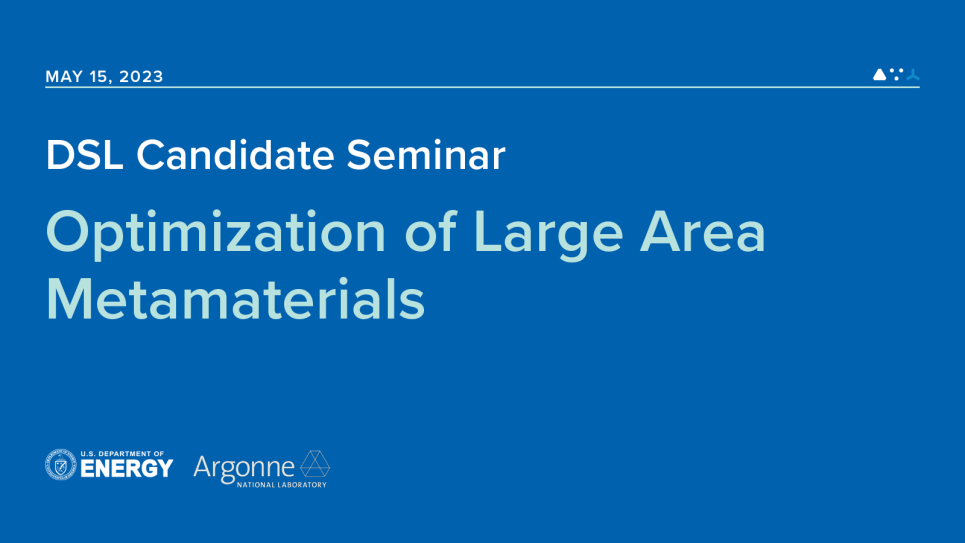
In recent years, quasi-periodic arrays of dielectric scatterers, also known as meta surfaces, have been shown to manipulate electromagnetic scattering with sub-wavelength spatial resolution. These devices have proven to be incredibly useful for reducing the form factor of conventional optical devices, as well as creating novel optical devices that are not possible to realize with conventional refractive optics. The design of these devices is however non-trivial. Conventional forward design methods are useful for generating only a small subset of possible designs. More complicated designs as well as optimization of existing forward designs requires solving the full-wave Maxwell's equations many times to converge to an optimal device design. This presentation outlines three main advancements in computational electromagnetics for the design of meta surfaces. Firstly, we extend the Generalized Multi-sphere Mie Scattering (GMMT) method to ellipsoidal scatterers. This method solves Maxwell's equations and computes gradients with respect to geometric design parameters of meta surfaces composed of ellipsoidal, dielectric scatterers analytically. We use this method to fully inverse design a meta surface lens, a meta-grating that focuses light at different focal planes for different polarizations and improve the efficiency of a forward designed lens from $25.59\%$ to $32.00\%$. The second method is a data-driven method that predicts electromagnetic fields from wavelength-scale cylindrical pillars, obtaining a low-dimensional representation of the data via the singular value decomposition. This method fits a differentiable neural-network model of the input geometries and configurations of the meta surface scatterers to the low-dimensional representations of the output field. This framework was used to design a device that produces an annular focal spot for the $\lambda=400nm$ wavelength, and a focal spot for the $\lambda=633nm$ wavelength. Finally, we present a data-free machine learning framework for predicting electromagnetic field responses from meta surfaces by using physics informed neural networks. This method predicts full fields from 1D meta-gratings by a neural network that is trained by minimizing the residual norm of the Maxwell operator. We use this technique to design a set of large area $(1mm)$ meta-lenses exhibiting higher efficiency than the ones designed under the local phase approximation. We validate our designs experimentally and see a maximum intensity improvement of up to $53\%$.
To add to calendar:
Click on: https://wordpress.cels.anl.gov/cels-seminars/
Enter your credentials.
Search for your seminar
Click “Add to calendar”