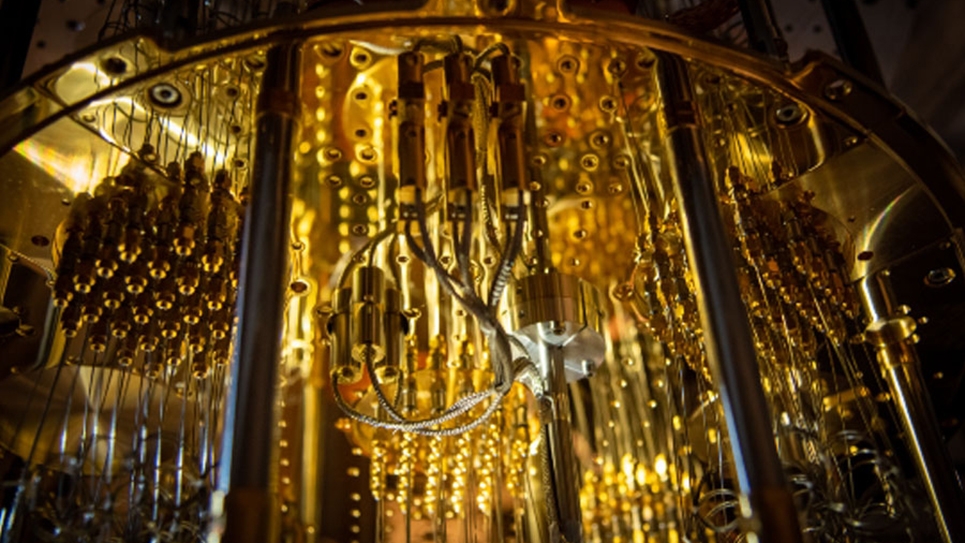
The absence of closed-form analytical models in many scientific problems makes system design, optimization, and control challenging. Reinforcement learning (RL), which is a machine learning (ML) paradigm for data-driven sequential decision making, enables holistic system design and control, can harness nonlocal regularities of noisy control trajectories, and facilitates transfer learning between tasks. In the first part of the talk, I will present my research in the development of RL-based methods for optimizing the variational parameters of the Quantum Approximate Optimization Algorithm (QAOA) to approximately solve combinatorial problems. In the second part of the talk, I will present my ongoing research to develop RL and ML algorithms to optimize future 5G wireless access networks with multiple objectives and constraints in support of a massive number of Internet-of-Things (IoT) devices. I will conclude the talk by highlighting some challenges pertaining to the integration of RL in scientific applications, and motivate my future research directions to facilitate this integration.
Please use this link to attend the virtual seminar: