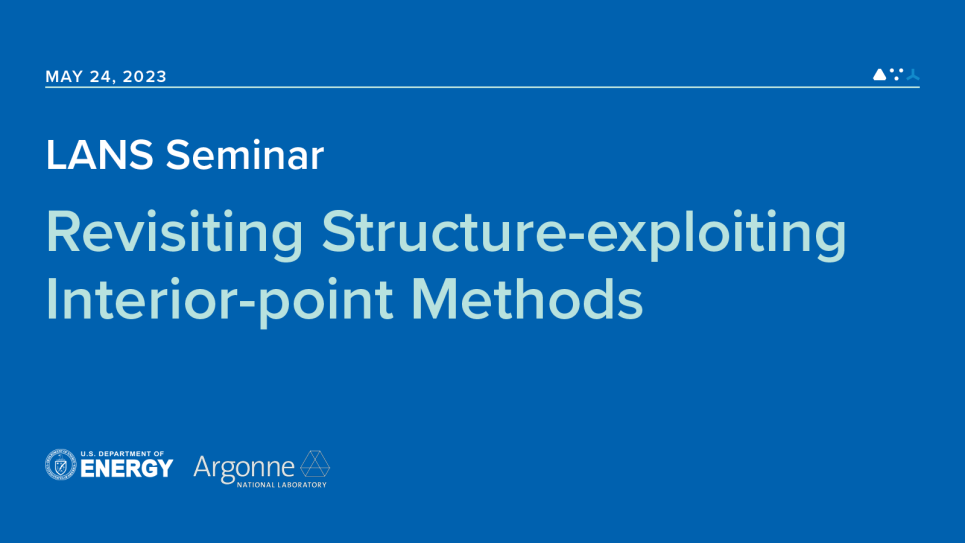
How far should you exploit the structure of your problem in nonlinear optimization? By design, many engineering problems are constrained by physical equations, leading to stringent restriction on the search-space. Hence, one can exploit the structure of the physical equations to split the optimization variables in two distinct sets, by isolating the independent variables (=degrees of freedom) from the dependent variables. If the number of degrees of freedom is small, we can drastically reduce the dimension of the problem by using a reduced-space approach, where we optimize only with relation to the independent variables. Dommel and Tinney have shown in 1968 that such reduced-space approach directly applies to the static optimal power flow problem (OPF), when one looks at optimizing the dispatch of generators in a power grid while satisfying the static (nonlinear) power flow equations. In that particular case, the reduced-space is directly associated to the manifold induced by the power flow equations. Incidentally, this approach has fallen out of flavor in the 1990s, with the development of mature sparse linear solvers able to solve frontally large-scale optimization problems. In this talk, we revisit the seminal method of Dommel and Tinney, with a modern glance. We exhibit the link existing between the reduced-space OPF approach and the classical OPF formulation. We show that by accelerating the computation of the reduced gradient and the reduced Hessian on GPUs, the method of Dommel and Tinney compares favorably with state-of-the-art interior-point solvers. We detail an extension of the method to solve stochastic and security-constrained OPF, interpreted here as two-stage stochastic nonlinear programs. We finish the talk by discussing potential extensions to transient-stability OPF, where this time the physical equations are encoded by a differential algebraic equations (DAE).
Bio: François Pacaud is a computational mathematician working in numerical optimization methods. Between 2020 and 2022, he was a postdoctoral appointee at Argonne National Lab, in the Mathematics and Computer Science (MCS) division. He is now an assistant professor at Mines Paris - PSL, France.
See all upcoming talks at https://www.anl.gov/mcs/lans-seminars