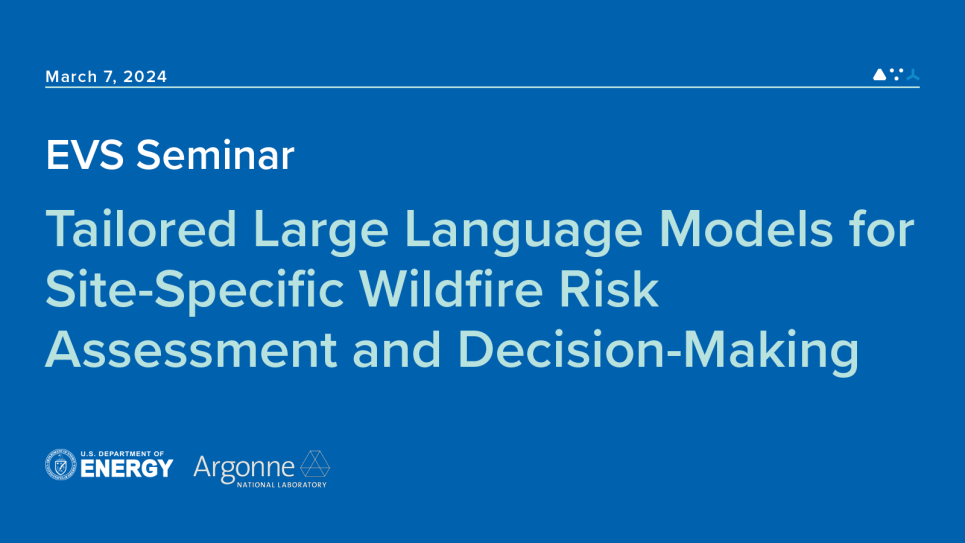
Climate projections provided by both public and private agencies often lack the necessary contextual information and require a considerable level of technical expertise. This complexity significantly hampers their utility for conducting detailed, site-specific analyses of future climate risks and making informed decisions. In this talk, we will demonstrate the development of specialized large language models (LLMs) designed to bridge this gap for wildfire analysis within the broader context of climate change. We prototyped WildfireGPT, an LLM agent specifically focused on wildfire analysis, designed to actively engage with users and transform their wildfire-related inquiries into actionable insights. We enhance the model by incorporating additional context, such as downscaled climate data projections from Argonne National Laboratory's Climate Risk and Resilience (ClimRR) portal and the extensive knowledge embedded within the scientific literature. This enables WildfireGPT to deliver detailed, user-specific insights on wildfire risks and support a diverse set of end-users, including engineers, urban planners, emergency managers, and infrastructure operators.
Bio: Tanwi is an Assistant Computer Science Specialist in the Mathematics and Computer Science Division at Argonne. Prior to this role, she was a Postdoctoral Appointee in the same division. Her current research focuses on spatiotemporal modeling, scalable data-efficient deep learning, foundation models, uncertainty quantification, and large-scale machine learning on high-performance computing systems. Before joining Argonne, Tanwi served as a Senior Data Scientist at General Electric. She earned her Ph.D. in Computer Science from the Indian Institute of Technology in Kharagpur, India.