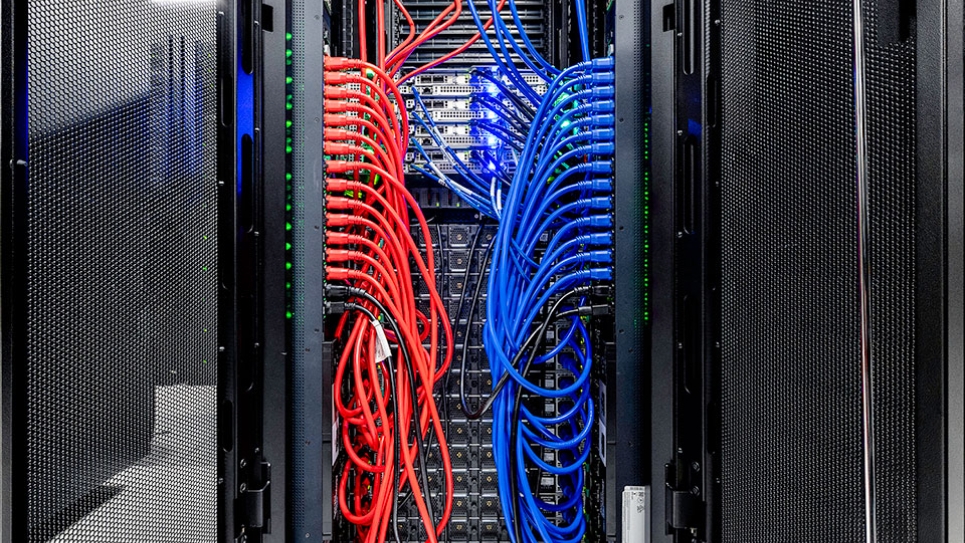
The ALCF AI Testbed's Graphcore Bow Pod64 system. (Image by Argonne National Laboratory)
Available to researchers worldwide, the ALCF AI Testbed has added a new Graphcore system along with upgraded Cerebras and SambaNova machines.
The Argonne Leadership Computing Facility’s (ALCF) AI Testbed housed at the U.S. Department of Energy’s Argonne National Laboratory is getting an update, ensuring that its global user community has continued access to leading-edge AI systems for scientific research.
The AI Testbed is a growing collection of some of the world’s most advanced AI accelerators designed to enable researchers to explore deep learning and machine learning workloads to advance AI for science. The systems are also helping the facility to gain a better understanding of how novel AI technologies can be integrated with traditional supercomputing systems powered by CPUs (central processing units) and GPUs (graphics processing units). The ALCF is a U.S. DOE Office of Science user facility at Argonne.
A major focus of the testbed is to help evaluate the usability and performance of machine learning-based high-performance computing applications on unique AI hardware. The ALCF’s AI platforms complement the facility’s current and next-generation supercomputers to provide a state-of-the-art environment that supports research at the intersection of AI, big data, and high-performance computing.
“Our upgraded AI Testbed systems provide unparalleled power and user-accessibility, enabling data-driven discoveries in critical DOE science domains. From advanced manufacturing and materials development to high energy particle physics, these systems will empower researchers to push the boundaries of scientific understanding and accelerate progress in both applied and basic research,” said Michael E. Papka, director of the ALCF and deputy associate laboratory director for Argonne’s Computing, Environment and Life Sciences directorate. “Whether requiring in-situ analysis of beamline imagery, rapid training of billion-parameter models, or learning-guided optimization for simulation runs, ALCF users can comprehensively leverage the latest AI technologies throughout the entirety of their research campaigns.”
This spring, the ALCF added a new Graphcore system along with upgraded Cerebras and SambaNova machines to its AI Testbed, providing access to powerful AI technologies to researchers worldwide.
The ALCF AI Testbed systems are available to the global research community; interested users can apply for free allocations on these machines at any time.
Deploying the Graphcore system
The 22-petaflop/s Graphcore Bow Pod64, an Intelligence Processing Unit (IPU) system, is the newest ALCF AI Testbed platform to be rolled out to the scientific community.
IPUs being well-suited for both common and specialized machine learning applications, these novel processors will help to facilitate the use of new AI techniques and model types.
Updates to the Cerebras and SambaNova systems
The addition of a Cerebras Wafer-Scale Cluster WSE-2, including the MemoryX system and SwarmX fabric, will optimize the Testbed’s existing Cerebras CS-2 system, enabling near-perfect linear scaling of large language models (LLMs). LLMS—deep learning models whose parameters number in the billions—stand to expand the possibilities of AI for science, but the distribution of such models across thousands of GPUs poses immense challenges, including sublinear performance.
“The Cerebras Wafer-Scale Cluster, by running data in parallel, has been demonstrated to achieve near-perfect linear scaling across millions of cores,” said Venkat Vishwanath, data science team lead at the ALCF. “This helps make extreme-scale AI substantially more manageable.”
The second-generation SambaNova DataScale system, meanwhile, comprising eight nodes for a total of 64 next-generation SN-30 Reconfigurable Datascale AI accelerators, enables a wider range of AI-for-science applications, making massive AI models and datasets more tractable to users.
Each accelerator is allocated a terabyte of memory, ideal for applications involving LLMs as well as high-resolution imaging from experimental facilities such as the Advanced Photon Source (APS), a DOE Office of Science user facility at Argonne.
ALCF users have already begun to leverage the AI Testbed systems in their research. Read about some of the efforts currently underway below.
Read the Graphcore Article - Argonne National Lab Makes BOW IPUS Available to Researchers Worldwide
Experimental data analysis
Mathew Cherukara, a computational scientist and group leader in Argonne’s X-ray Science division, is exploring the use of multiple testbed systems to accelerate and scale deep learning models—including for 3D and 2D imaging, as well as for grain mapping—to aid the analysis of X-ray data obtained at the APS.
“Several APS beamlines routinely collect data at rates exceeding a gigabyte per second, and these data need to be analyzed on millisecond timescales,” he explained.
Cherukara is using the ALCF’s Cerebras system for fast training (including training performed on live instrument data), while the SambaNova machine will help train models—too large to run on a single GPU—to generate improved 3D images from X-ray data. He noted that related work is being performed to explore the use of the AI Testbed’s Groq system in fast-inference applications.
Porting models in use at the APS (PtychoNN, BraggNN, and AutoPhaseNN) to the ALCF’s AI systems has yielded promising initial results, including a number of speedups over traditional supercomputers. ALCF and vendor software teams are collaborating with Cherukara’s team to achieve further advances.
“Our end goal is to use the testbeds in production. Every year more than 5,000 scientists from across the country and world use the unique capabilities that the APS provides,” Cherukara said. “These users need fast, accurate data analysis performed in sync with the experiment and the AI Testbed systems have the potential to address those needs by providing fast, scalable deep learning model training and inference.”
Neural networks
Graph neural networks (GNNs) are powerful machine learning tools that can process and learn from data represented as graphs. GNNs are being used for research in several areas, including molecular design, financial data, and social networks. Filippo Simini, an ALCF computer scientist, is working to compare the performance of GNN models across multiple AI accelerators, including Graphcore IPUs.
“With a focus on inference, my team is examining which GNN-specific operators or kernels, as a result of increasing numbers of parameters or batch sizes, can create computational bottlenecks that affect overall runtime,” he said.
COVID-19 research
Arvind Ramanathan, a computational biologist in Argonne’s Data Science and Learning division, and his team relied on the AI Testbed when using LLMs to discover SARS-CoV-2 variants. Their workflow leveraged the Cerebras CS-2 and Wafer-Scale Cluster alongside GPU-accelerated systems including the ALCF’s Polaris supercomputer.
He emphasized that one of the critical problems to overcome in his work was how to manage extensive genomic sequences, the size of which can overwhelm many computing systems when establishing foundation models. The learning-optimized architecture of the Cerebras systems was key for accelerating the training process. The team’s research resulted in the 2022 Gordon Bell Award Special Prize for COVID-19 Research.
Molecular simulations
Logan Ward, a computational scientist in Argonne’s Data Science and Learning division, has been leading efforts to run an application that carries out two types of computations for research into potential battery materials. In the first type of computation, his team runs physics simulations of molecules under redox—that is, they calculate how much energy molecules can store when electrically charged. In the second, they train a machine learning model that predicts that energy quantity.
“Our application ultimately combines the two computations together,” Ward said. “It uses the trained machine learning model to predict the outcomes of the redox calculations, such that it can run the calculations that identify molecules with the desired capacity for energy storage.”
His work seeks to make this process as efficient as possible.
He detailed some of the advantages gained by using the system: “Graphcore enables shortened latency when cycling between the execution of a new calculation that yields additional training data and when that model is used to select the next calculation.”
Existing workflows can be leveraged to jumpstart use of AI Testbed systems, and the ALCF’s expert staff is available to provide users with hands-on help to achieve the best possible results. For more information, including on how to obtain an allocation, please visit: https://www.alcf.anl.gov/alcf-ai-testbed
==========
The Argonne Leadership Computing Facility provides supercomputing capabilities to the scientific and engineering community to advance fundamental discovery and understanding in a broad range of disciplines. Supported by the U.S. Department of Energy’s (DOE’s) Office of Science, Advanced Scientific Computing Research (ASCR) program, the ALCF is one of two DOE Leadership Computing Facilities in the nation dedicated to open science.
Argonne National Laboratory seeks solutions to pressing national problems in science and technology. The nation's first national laboratory, Argonne conducts leading-edge basic and applied scientific research in virtually every scientific discipline. Argonne researchers work closely with researchers from hundreds of companies, universities, and federal, state and municipal agencies to help them solve their specific problems, advance America's scientific leadership and prepare the nation for a better future. With employees from more than 60 nations, Argonne is managed by UChicago Argonne, LLC for the U.S. Department of Energy's Office of Science.
The U.S. Department of Energy's Office of Science is the single largest supporter of basic research in the physical sciences in the United States and is working to address some of the most pressing challenges of our time. For more information, visit https://energy.gov/science