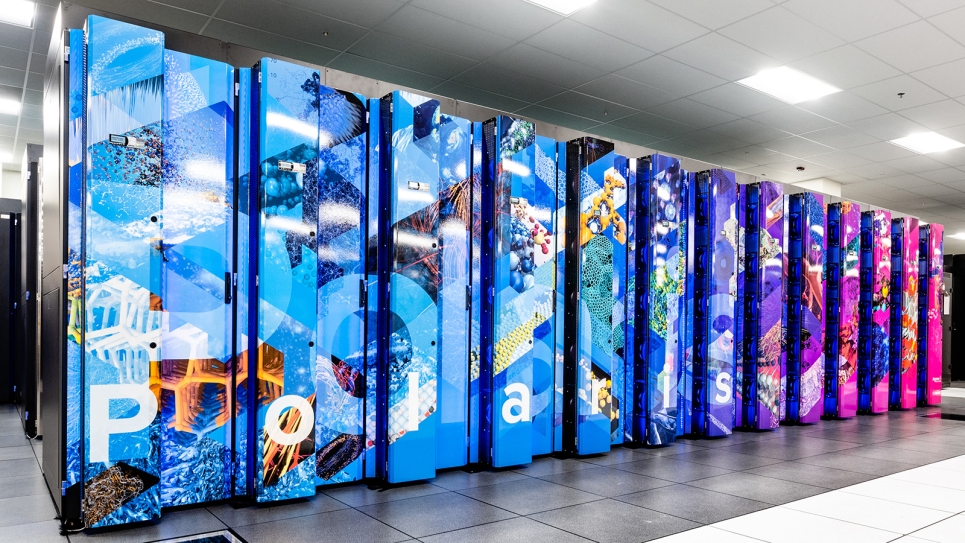
Argonne researchers announced storage performance benchmarks for the ALCF's Polaris supercomputer that showcase its capability for efficient state-of-the-art AI operations. Image: Argonne National Laboratory
As part of the new MLPerf storage performance benchmarks, ALCF researchers demonstrated the capabilities of its Polaris supercomputer and Eagle file system in training machine learning workloads.
Researchers from Argonne National Laboratory have demonstrated new storage performance benchmarks for the Polaris supercomputer housed at the Argonne Leadership Computing Facility (ALCF), a U.S. Department of Energy (DOE) Office of Science user facility.
As part of the first-ever release of storage performance benchmarks from the open AI engineering consortium MLCommons, Argonne researchers led by ALCF computer scientist Huihuo Zheng—who co-chaired the evaluation—announced the results of their assessment of Polaris and its supporting file system, the Eagle Lustre file system. The benchmarks, which were created through a collaboration of AI leaders in industry and academia, measure storage performance for machine learning (ML) training workloads.
Performance was assessed using two distinct MLPerf Storage AI workloads, UNet3D and Bert. Polaris, developed in collaboration with Hewlett Packard Enterprise (HPE), delivers 44 double-precision tensor flops and comprises 560 nodes interconnected via HPE Slingshot. Each node has four NVIDIA A100 GPU accelerator and two 1.6 terabyte NVMe drives. Eagle is a Lustre parallel file system residing on an HPE ClusterStor E1000 platform, offering 100 petabytes of usable capacity across 8480 disk drives. It has 160 object storage targets and 40 metadata targets, with an aggregate transfer rate of 659 gigabytes per second (GB/s).
The storage benchmarks were achieved on Polaris by running datasets hosted on both the Eagle Lustre file system and node-local solid-state drives (SSDs), so as to emulate the behavior of users executing AI workloads. The announced results demonstrate a remarkable linear performance increase in I/O throughput for both UNet3D and Bert as the workloads were scaled to 2048 accelerators. Polaris’s efficient I/O handling enables data transfers to overlap with computation, achieving an effective accelerator utilization rate of nearly 100 percent.
The I/O-intensive UNet3D achieved a peak throughput of 200 GB/s with the Eagle file system; leveraging the node-local NVMe SSDs, the researchers observed throughput of 800 GB/s. The less-intensive Bert workload demonstrated the same remarkable scaling pattern, showcasing the capability of ALCF systems for efficient state-of-the-art AI operations.
For more information on the MLCommons benchmarks, please visit: https://mlcommons.org/en/news/mlperf-inference-storage-q323/