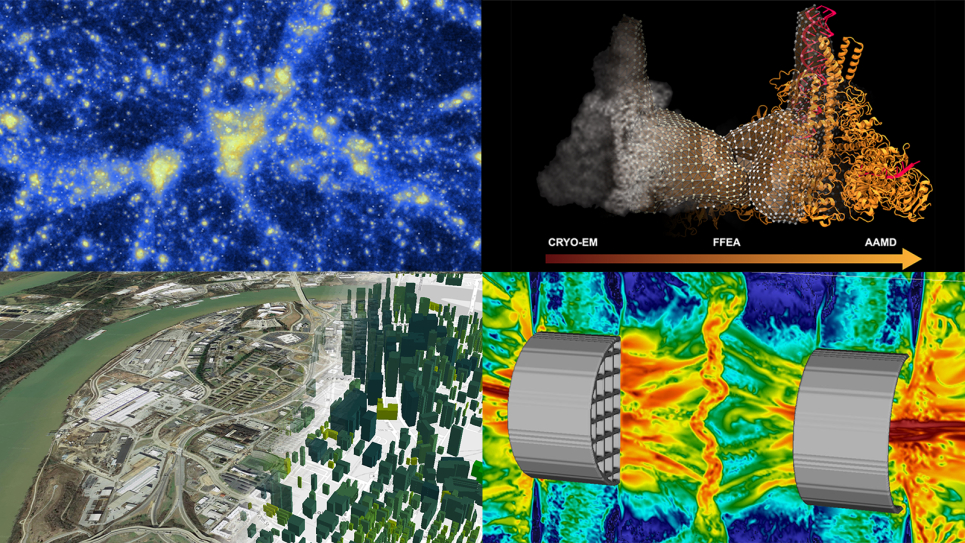
The ALCF user community continued to push the boundaries of scientific computing, producing groundbreaking studies in areas ranging from cosmology to COVID-19 research.
In 2021, scientists from across the world used supercomputers at the U.S. Department of Energy’s (DOE) Argonne National Laboratory to produce a number of impactful studies and publications, accelerating discovery and innovation in a wide range of research areas.
From modeling the energy use of the nation’s buildings to advancing our understanding of SARS-CoV-2, projects supported by the Argonne Leadership Computing Facility (ALCF) continued to push the state of the art in using large-scale simulations, artificial intelligence (AI), and data analysis techniques for scientific research.
As 2021 draws to a close, we take a look back at some of the groundbreaking research campaigns carried out by the ALCF user community over the past year. For more examples of the scientific advances enabled by ALCF computing resources, check out the 2021 ALCF Science Report.
SARS-CoV-2, the virus that causes COVID-19, propagates via a precisely coordinated process known as the replication-transcription complex to reproduce at high speed when it invades a host’s cells. A multi-institutional research team leveraged a combination of AI and high-performance computing (HPC) technologies, including ALCF’s Theta supercomputer and AI Testbed, to explore the intricacies of how the virus reproduces itself. To do so, the team employed a hierarchical AI framework running on Balsam, a distributed workflow manager developed at ALCF, across four of the nation’s top supercomputing facilities: ALCF, Oak Ridge Leadership Computing Facility (OLCF), National Energy Research Scientific Computing Center (NERSC), and Texas Advanced Computing Center. The team’s computations helped fill in the blanks that could not be captured with cryo-electron microscopy techniques, leading to a better understanding of the inner workings of the SARS-CoV2 replication process. The study was recognized with a finalist nomination for the Association of Computing Machinery (ACM) Gordon Bell Special Prize for High Performance Computing-Based COVID-19 Research. Watch the team’s presentation at SC21 here. The ALCF, OLCF and NERSC are DOE Office of Science user facilities. The team’s research was also supported by DOE’s National Virtual Biotechnology Laboratory and the COVID-19 HPC Consortium.
To help improve the efficiency of deep learning-driven research, a team from the ALCF and the Illinois Institute of Technology (IIT) developed a new benchmark, named DLIO, to help researchers identify bottlenecks that hinder data input/output (I/O) performance in deep learning applications. The team used DLIO to evaluate project workloads from DOE’s Exascale Computing Project, the ALCF’s Aurora Early Science Program, and the ALCF Data Science Program to understand the typical I/O patterns of various scientific deep learning applications that run on leadership computing resources. While many applications use scientific data formats that are not well-supported by deep learning frameworks, the team leveraged DLIO to identify bottlenecks and guide optimizations that led to a six-fold improvement in efficiency and time to solution for some of the target applications. The researchers were awarded best paper for their work with DLIO at the Institute of Electrical and Electronics Engineers (IEEE)/Association for Computing Machinery (ACM) International Symposium on Cluster, Cloud and Internet Computing (CCGrid).
A team from Oak Ridge National Laboratory used the ALCF’s Theta supercomputer for a simulation campaign that assessed energy use across more than 178,000 buildings in Chattanooga, Tennessee. The effort, which will continue with a 2022 INCITE project, is part of a larger goal to model the energy consumption of all of the nation’s 129 million buildings. Creating an energy picture of a large network of buildings, rather than looking at just one building or even several hundred, can illuminate areas of opportunity for planning the most effective energy-saving measures. The Oak Ridge team is partnering with companies to make their building energy models and analysis publicly available and free to access, with the aim of stimulating private sector activity toward more grid-aware energy efficiency alternatives.
Knowing where drug-binding sites are located on target proteins is critical to drug discovery efforts. To help address this challenge for SARS-CoV-2, researchers from Johns Hopkins School of Medicine leveraged ALCF resources in the development of a novel approach that combines molecular dynamics simulations and a machine learning method called TACTICS (Trajectory-based Analysis of Conformations To Identify Cryptic Sites) to predict drug binding sites. As proof-of-principle, the team employed TACTICS to analyze simulations of the SARS-CoV-2 proteins. Their approach successfully identified known druggable sites and predicted the locations of sites not previously observed in experimentally determined structures, providing new opportunities for drug development. Their findings were published in the Journal of Chemical Information and Modeling.
Using ALCF computing resources, an international team of researchers carried out simulations to design groundbreaking laser experiments that shed light on the mysterious origins of cosmic magnetic fields. The team’s simulations helped determine the parameters needed to recreate and study a phenomenon known as turbulent dynamo in a laboratory for the first time via experiments conducted at the University of Rochester’s Omega Laser Facility at the Laboratory for Laser Energetics. Turbulent dynamo, a mechanism thought to play an important role in generating the magnetic fields present in planets, stars, galaxies, and galaxy clusters, had previously been studied only through theoretical calculations and numerical simulations. Their findings were detailed in a paper published in the Proceedings of the National Academy of Sciences.
Researchers from DOE’s Princeton Plasma Physics Laboratory (PPPL) are using leadership computing resources at the ALCF and OLCF to address some of the most pressing plasma physics questions facing future fusion energy devices, such as ITER, the world's largest magnetic fusion experiment under construction in France. One of the most important requirements for ITER is the tokamak’s divertor, a material structure engineered to remove exhaust heat from the reactor’s vacuum vessel. The heat-load width of the divertor is the width along the reactor’s inner walls that will sustain repeated hot exhaust particles coming in contact with it. Leveraging large-scale simulations and machine learning techniques, the PPPL team developed a new predictive scaling formula to help researchers understand the heat-load width requirements of the future ITER device. Using this approach, the researchers demonstrated that ITER’s divertor heat-load width at full power would be more than six times wider than was expected in the current trend of tokamaks. The team’s results were published in Physics of Plasmas.
A team of researchers from Argonne and Oak Ridge National Laboratories used ALCF and OLCF supercomputers to accurately calculate the geometry of a complex 2D nanomaterial called germanium selenide (GeSe) for the first time, shedding light on a promising material for applications such as solar cells and photodetectors. Employing a new quantum Monte Carlo-based algorithm, the researchers discovered that the properties of a single layer of GeSe are extremely dependent on atomic arrangement. Scientists can change how materials such as GeSe emit and absorb light by modifying their geometries, providing an avenue to advance the design and discovery of novel materials for targeted applications. The team’s research was published in Physical Review Materials.
As part of the ExaSMR project within DOE’s Exascale Computing Project, researchers used Argonne supercomputing resources to complete the first ever full-core pin-resolved computational fluid dynamics (CFD) model of a small modular reactor (SMR). The ultimate research objective of ExaSMR is to carry out full-core multiphysics simulations that couple both CFD and neutron dynamics on DOE’s upcoming exascale supercomputers. Instead of creating a computational grid that resolves all the local geometric details, the researchers developed a framework that can improve the accuracy of simulations of thermal-fluid behavior inside a SMR core without sacrificing computational efficiency. Their work represents a significant advancement in capability for CFD modeling of nuclear reactors and demonstrates the benefits of further integrating high-fidelity numerical simulations in actual engineering designs. The team’s research was published in Nuclear Engineering and Design.
Before the ALCF’s previous-generation Mira supercomputer was retired, Argonne researchers used the system to perform one of the world’s largest cosmological simulations. The massive simulation, called the Last Journey, evolved more than 1.24 trillion particles to follow the distribution of mass across the universe over time — in other words, how gravity causes a mysterious invisible substance called dark matter to clump together to form larger-scale structures known as halos, within which galaxies form and evolve. The Last Journey simulation provides data for ongoing and upcoming cosmological surveys to use when comparing observations or drawing conclusions about a host of topics. This could lead to insights into several outstanding cosmological mysteries, including the role of dark matter and dark energy in the evolution of the universe. In 2021, the team used the simulation results to publish two papers in the Astrophysical Journal: “The Last Journey. I. An Extreme-scale Simulation on the Mira Supercomputer” and “The Last Journey. II. SMACC–Subhalo Mass-loss Analysis using Core Catalogs.”
With the aid of ALCF computing resources, researchers from the University of Southern California are combining advanced AI techniques with leadership-scale quantum dynamics simulations and experimental data to explore new computational methods for materials discovery and development. In 2021, the team published multiple papers that highlight their use of emerging AI methods to identify promising material compositions and phases. In a study published in Physical Review Letters, they employed neural networks to investigate the dielectric constant and its temperature dependence for liquid water. Their scalable method, which is applicable to a range of different materials and systems, computed dielectric constants that were in good agreement with experimental data.
The team used reinforcement learning (RL) for two additional studies published in npj Computational Materials. In one paper, the researchers deployed an autonomous RL agent to predict the optimal synthesis conditions for molybdenum disulfide ( MoS2), a 2D quantum material, using chemical vapor deposition. In the other study, they successfully used RL to generate a wide range of highly stretchable MoS2 kirigami structures from an extremely large search space consisting of millions of structures. Ultimately, the team’s research is providing insights that will help advance efforts to discover and synthesize novel materials for targeted applications.
==========
The Argonne Leadership Computing Facility provides supercomputing capabilities to the scientific and engineering community to advance fundamental discovery and understanding in a broad range of disciplines. Supported by the U.S. Department of Energy’s (DOE’s) Office of Science, Advanced Scientific Computing Research (ASCR) program, the ALCF is one of two DOE Leadership Computing Facilities in the nation dedicated to open science.
Argonne National Laboratory seeks solutions to pressing national problems in science and technology. The nation’s first national laboratory, Argonne conducts leading-edge basic and applied scientific research in virtually every scientific discipline. Argonne researchers work closely with researchers from hundreds of companies, universities, and federal, state and municipal agencies to help them solve their specific problems, advance America’s scientific leadership and prepare the nation for a better future. With employees from more than 60 nations, Argonne is managed by UChicago Argonne, LLC for the U.S. Department of Energy’s Office of Science.
The U.S. Department of Energy’s Office of Science is the single largest supporter of basic research in the physical sciences in the United States and is working to address some of the most pressing challenges of our time. For more information, visit https://energy.gov/science.