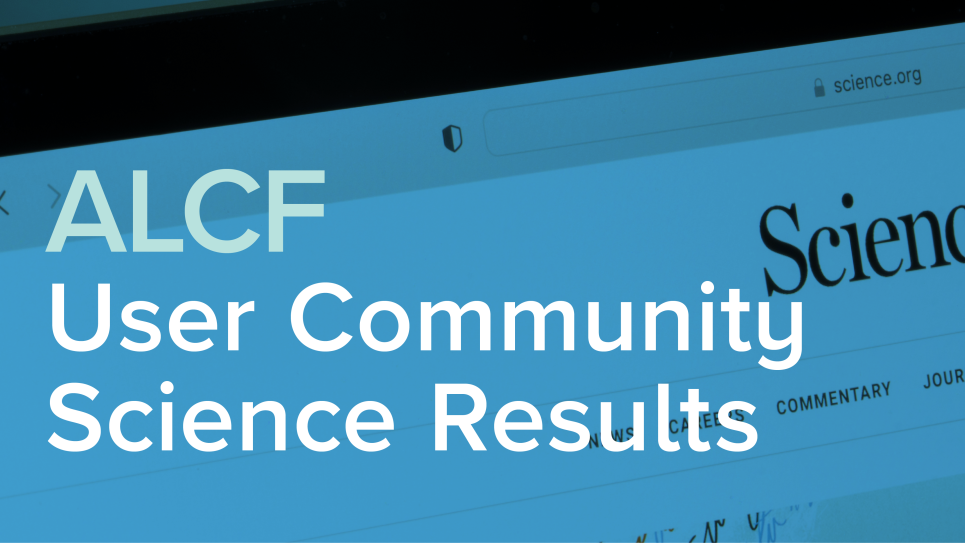
ALCF User Community Science Results
Using supercomputing resources at the ALCF, researchers produce a number of impactful studies and publications across scientific domains.
The ALCF user community continually pushes the boundaries of scientific computing, producing groundbreaking studies in areas ranging from chemistry and engineering to physics and materials science. Below, we highlight some of the recent results published by ALCF users in peer-reviewed journals.
Evaluation of Precipitation Across the Contiguous United States, Alaska, and Puerto Rico in Multi-Decadal Convection-Permitting Simulations, Scientific Reports (January 2024), Springer Nature.
This study is an early effort to generate a multi-decadal convection-permitting regional climate dataset that covers nearly the entire North American continent. Using ALCF leadership-computing resources, the researchers assessed a 20-year dynamically downscaled regional climate simulation at a 4 km spatial resolution with explicit convection across the contiguous United States (CONUS), Alaska, and Puerto Rico. Specifically, they evaluated the model’s performance in representing mean, 95th percentile, and extreme precipitation across regions. Their findings indicate that when compared with ERA5 reanalysis, the forcing data, convection-permitting simulation improves representations of seasonal, 95th percentile, and extreme precipitation over a large portion of the CONUS, Alaska, and Puerto Rico, particularly in areas where precipitation is heaviest. The simulation adds value over its forcing data (ERA5) in up to 53% of all grid cells in the CONUS, 68.8% in Alaska, and 84.0% in Puerto Rico. It is important to note that, however, despite improvements, model errors in Puerto Rico remain large. Similar improvements are observed in extreme indices, including consecutive dry days, maximum 5 days precipitation, and extreme precipitation. Analysis of the diurnal cycle of mean hourly precipitation suggests that representations of convective processes—including onset, dissipation, suppression, downstream propagation, and local circulation—improved overall.
Isochronic Development of Cortical Synapses in Primates and Mice, Nature Communications (December 2023), Springer Nature.
The neotenous, or delayed, development of primate neurons, particularly human ones, is thought to underlie primate-specific abilities like cognition. The authors tested whether synaptic development follows suit—would synapses, in absolute time, develop slower in longer-lived, highly cognitive species like non-human primates than in shorter-lived species with less human-like cognitive abilities, e.g., the mouse? Instead, the study finds that excitatory and inhibitory synapses in the male Mus musculus (mouse) and Rhesus macaque (primate) cortex form at similar rates, at similar times after birth. Primate excitatory and inhibitory synapses and mouse excitatory synapses also prune in such an isochronic fashion. Mouse inhibitory synapses are the lone exception, which are not pruned and instead continuously added throughout life. The monotony of synaptic development clocks across species with disparate lifespans, experiences, and cognitive abilities argues that such programs are likely orchestrated by genetic events rather than experience. The researchers used ALCF supercomputers to scale and parallelize a custom algorithmic pipeline for creating 3D electron microscopy volumes, tracing neuronal processes and identifying their connections, and incorporating human error checking
Explainability and Extrapolation of Machine Learning Models for Predicting the Glass Transition Temperature of Polymers, Journal of Polymer Science (December 2023), John Wiley and Sons.
Machine learning (ML) offers promising tools to develop surrogate models for polymers' structure–property relations. Surrogate models can be built upon existing polymer data and are useful for rapidly predicting the properties of unknown polymers. The accuracy of such ML models appears to depend on the feature space representation of polymers, the range of training data, and learning algorithms. In this paper the authors establish connections between these factors for predicting the glass transition temperature (Tg) of polymers. Their analysis suggests linear models with fewer fitting parameters are as accurate as nonlinear models with many hidden and unexplainable parameters. Also, the performance of a monomer topology-based ML model is found to be qualitatively identical to that of a physicochemical descriptor-based ML model. The authors find that the ML models' performance in the extrapolative region is enhanced as the property range of the training data increases. Moreover, they establish new Tg – polymer chemistry correlations via ML. Their work, carried out with ALCF supercomputing resources, illustrates how ML can advance the fundamental understanding of polymer structure–property correlations and its efficacy for extrapolation problems.
Gravitational Form Factors of the Pion from Lattice QCD, Physical Review D (December 2023), APS.
The authors leverage DOE resources, including ALCF supercomputers, to compute the two gravitational form factors of the pion as functions of the momentum transfer in the kinematic region on a lattice quantum chromodynamics (QCD) ensemble.