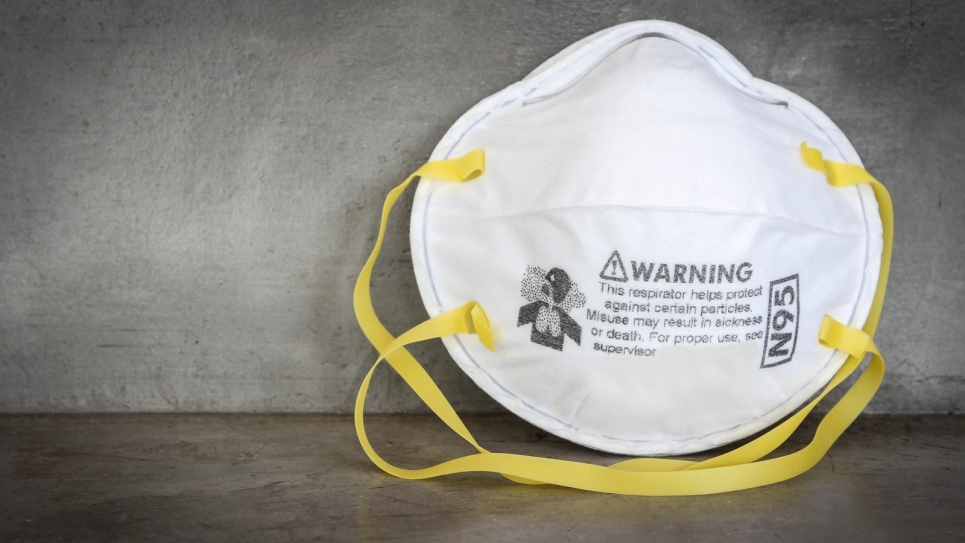
Scientists at Argonne have partnered with 3M in a computational effort to reduce energy costs of producing melt-blown nonwoven materials like the ones found in medical masks. (Image by Shutterstock/Smile Studio AP.)
Using AI and ALCF supercomputing resources, scientists from Argonne and 3M are collaborating to improve the energy efficiency of a manufacturing process used produce melt-blown nonwoven materials.
Making the countless numbers of N95 masks that have protected millions of Americans from COVID requires a process that not only demands attention to detail but also requires lots of energy. Many of the materials in these masks are produced by a technique called melt blowing, in which tiny plastic fibers are spun at high temperatures that necessitate the use of a lot of energy. The process is also used for other products like furnace filters, coffee filters and diapers.
Thanks to a new computational effort being pioneered by the U.S. Department of Energy’s (DOE) Argonne National Laboratory in conjunction with 3M and supported by the DOE’S High Performance Computing for Energy Innovation (HPC4EI) program, researchers are finding new ways to dramatically reduce the amount of energy required for melt blowing the materials needed in N95 masks and other applications.
Currently, the process used to create a nozzle to spin nonwoven materials produces a very high-quality product, but it is quite energy intensive. Approximately 300,000 tons of melt-blown materials are produced annually worldwide, requiring roughly 245 gigawatt-hours per year of energy, approximately the amount generated by a large solar farm. By using Argonne supercomputing resources to pair computational fluid dynamics simulations and machine-learning techniques, the Argonne and 3M collaboration sought to reduce energy consumption by 20% without compromising material quality.
The melt blowing process uses a die to extrude plastic at high temperatures. Finding a way to create identical plastic components at lower temperatures and pressures motivated the machine-learning search, said Argonne computational scientist Benjamin Blaiszik, an author of the study. “It’s kind of like we are trying to make a pizza in an oven — we’re trying to find the right dimensions, materials for our pizza stone, and cooking temperature using an algorithm to minimize the amount of energy used while keeping the taste the same,” he said.
By using simulations and machine learning, Argonne researchers can run hundreds or even thousands of use cases, an exponential improvement on prior work. “We have the ability to tweak things like the parameters for the die geometry,” Blaiszik said. “Our simulations will make it possible for someone to make an item at an actual industrial facility, and our computer can tell you about its potential for real-world applications.”
The simulations provide key insights into the process, a method to assess a combination of parameters that are used to generate data for the machine-learning algorithm. The machine-learning model can then be leveraged to ultimately converge on a design that can deliver the required energy savings.
Because the process of making a new nozzle is very expensive, the information gained from the machine-learning model can equip material manufacturers with a way to narrow down to a set of optimal designs. “Machine-learning-enhanced simulation is the best way of cheaply getting at the right combination of parameters like temperatures, material composition, and pressures for creating these materials at high quality with less energy,” Blaiszik said.
The initial model for the melt-blowing process was developed through a series of simulation runs performed on the Theta supercomputer at the Argonne Leadership Computing Facility (ALCF) with the computational fluid dynamics (CFD) software OpenFOAM and CONVERGE. The ALCF is a DOE Office of Science user facility located at Argonne.
==========
The Argonne Leadership Computing Facility provides supercomputing capabilities to the scientific and engineering community to advance fundamental discovery and understanding in a broad range of disciplines. Supported by the U.S. Department of Energy’s (DOE’s) Office of Science, Advanced Scientific Computing Research (ASCR) program, the ALCF is one of two DOE Leadership Computing Facilities in the nation dedicated to open science.
Argonne National Laboratory seeks solutions to pressing national problems in science and technology. The nation's first national laboratory, Argonne conducts leading-edge basic and applied scientific research in virtually every scientific discipline. Argonne researchers work closely with researchers from hundreds of companies, universities, and federal, state and municipal agencies to help them solve their specific problems, advance America's scientific leadership and prepare the nation for a better future. With employees from more than 60 nations, Argonne is managed by UChicago Argonne, LLC for the U.S. Department of Energy's Office of Science.
The U.S. Department of Energy's Office of Science is the single largest supporter of basic research in the physical sciences in the United States and is working to address some of the most pressing challenges of our time. For more information, visit https://energy.gov/science