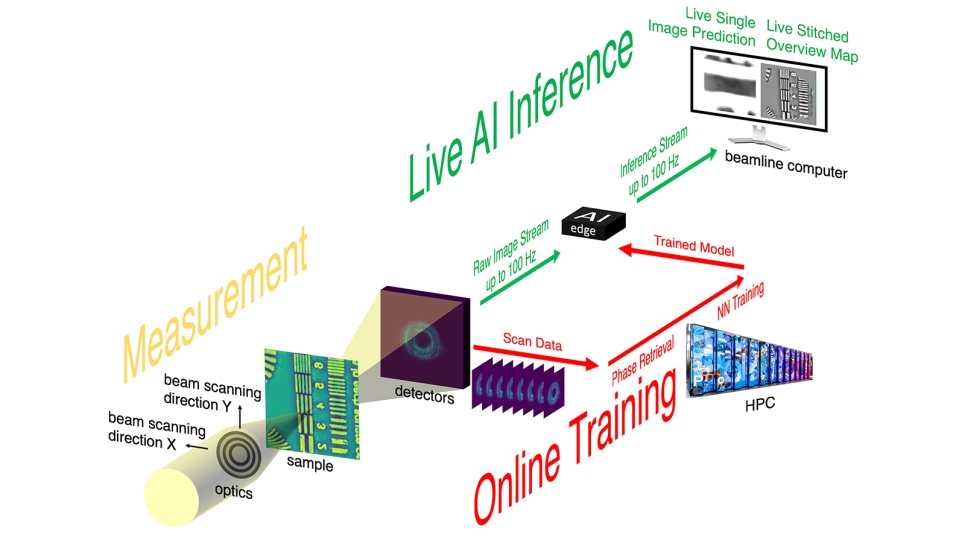
A schematic showing the experimental setup. (Image by Argonne National Laboratory.)
With help from ALCF computing resources, an Argonne team developed a new streaming ptychography technique that allows researchers to tweak experiments based on real-time data.
When scientists want to look at a tiny structure in a material, even one just a few atoms in size, they frequently turn to X-ray microscopy.
X-ray microscopes are advancing to the point where they are generating more data than scientists can hope to process efficiently, even with large supercomputers. As a result, researchers are looking for new techniques that can allow them to process data on the fly, which means analyzing data as it is collected and then feeding the results back into the experiment to ultimately create a pathway of autonomous discovery.
Scientists at the Advanced Photon Source (APS) at the U.S. Department of Energy’s (DOE) Argonne National Laboratory have recently developed a new method that incorporates machine learning — in the form of a neural network — into an X-ray microscopy technique. The new process enables researchers to take less time to sample their material and increases the rate of data processing by more than 100-fold while also reducing the amount of data collected by 25-fold. The APS is a DOE Office of Science user facility.
“The problem is that conventional means of analysis can’t keep up with data rates,” said Argonne group leader and computational scientist Mathew Cherukara, an author of the study. “And so we’re in this situation where you have these amazingly complex, extraordinary pieces of hardware, but we don’t have a means of analyzing all the data that they can produce.”
According to Cherukara, analyzing the data from these studies without a supercomputer could take days to weeks, and even with a supercomputer they could still take hours.
“The new neural network means that we can run many of these experiments in a few minutes at the full speed of the instrument,” he said.
Argonne group leader Antonino Miceli, another author of the study, noted that the ability to conduct these experiments quickly and adjust conditions spontaneously would allow scientists or autonomous instruments to make “split-second choices” about how to analyze the sample.
“If you don’t have the ability to analyze data on the fly, you wouldn’t be able to make these kinds of decisions,” he said.
The new technique could end up freeing time for more and better experiments at the APS, said Argonne physicist Tao Zhou, another author of the study.
“Most people who come to the APS, they travel, they come prepared for a week of experiments, and at the end of the week, they leave with their data, which they would analyze back home,” Zhou said. “If they find something interesting during the analysis that they want to do more measurements on, they typically have to wait for the next cycle of experiments at the APS. This technique essentially allows people to do the analysis in real time on the beamline, so if they see something new and interesting in the sample that they don’t anticipate, they can be ready and able to adapt almost immediately.”
The new technique is called streaming ptychography (ty-KAH-grah-fee). The streaming part works much like a video-streaming app like Netflix, except that it can make changes to the experiment itself as scientists, or other machine learning agents, react to what they are seeing. Imagine a house cat adjusting its leap as it tries to catch the red dot of a laser pointer and you have the idea. As the data is analyzed in near-real time, the focus of the experiment can be shifted to zero in on interesting phenomena as they are spotted.
“We’re gaining the ability to analyze our data while it’s being generated. The AI is learning as the experiment is progressing,” Cherukara said.
“Having this embedded computing close to the beamline allows us to do on-the-fly adjustments immediately while the experiment is in progress without having to send data back to a cloud or supercomputing cluster,” added Anakha Babu, a former Argonne postdoctoral researcher who is currently a researcher at KLA-Tencor and another author of the study. “This kind of setup can have uses beyond ptychography as well, in a wide range of experiments where adjustments based on the data can be needed in real time.”
Ptychography, an imaging technique widely used in X-ray, optical and electron microscopy, has long been recognized for its ability to provide high-resolution imaging of centimeter-sized objects with minimal sample preparation. However, the traditional methods used for image processing can be slow and computationally intensive, hindering real-time imaging.
The streaming process worked like this: The developers deployed high performance computers at the Argonne Leadership Computing Facility (ALCF) to carry out the first round of computationally intensive phase retrieval calculations from diffraction patterns generated by X-ray beams. These measurements were then used to train a neural network that could then more quickly perform accurate similar calculations at lower computational cost closer to the beamline. The ALCF is also a DOE Office of Science user facility.
“You need to make sure that your neural network is trained well,” said Argonne computer scientist Tekin Bicer, another author of the study. “As the training of the model runs during the experiment, it produces estimates that are close to what you would have achieved on a supercomputer at a fraction of the computational cost, vastly reducing lag time.”
Bicer explained that the initial training of the neural network doesn’t have to take long.
“When you first turn on the instrument, you can’t use a neural network because it hasn’t been trained yet,” he said. “But then as soon as you’ve got a little bit of data from the conventional analysis, you can start using the neural network.”
By using machine learning on individual X-ray diffraction patterns, the workflow eliminates the need for the usual stringent overlapping sampling constraints typical of ptychography, also greatly reducing the required beam dose. This breakthrough reduces the risk of sample damage, making it suitable for imaging delicate materials.
The neural network could also be applied to electron and optical microscopes, Cherukara explained.
“The most advanced microscopes in the world are no longer going to be held up by lack of analysis capabilities, and they’ll be able to operate at their full potential,” he said.
A paper based on the study appeared in Nature Communications.
The work was funded by the DOE Office of Science, Office of Basic Energy Sciences and an Argonne Laboratory-Directed Research and Development grant. The work used Argonne’s Hard X-Ray Nanoprobe beamline, which is co-operated by the APS and Argonne’s Center for Nanoscale Materials, another DOE Office of Science user facility.