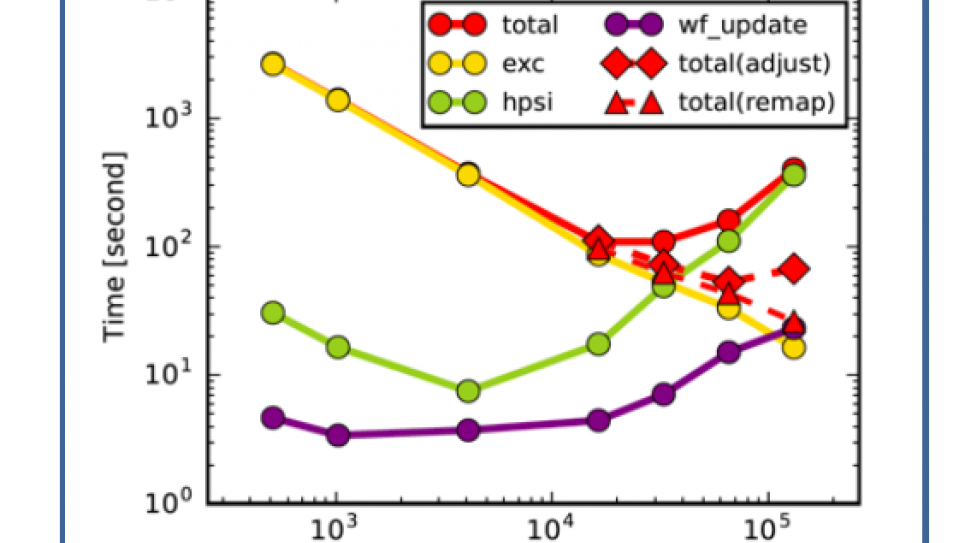
The strong scaling of Qbox hybrid-DFT calculations was extended on Theta by optimal data remapping methods (red triangles). An example is shown for solid (SiC)256 using the PBE0 functional.
Numerical simulations of the physical processes that occur in materials when they are excited with light are essential for the understanding and rational design of broad classes of materials. This project seeks to develop an AI-informed computational framework that integrates machine learning into existing workflows, thereby enabling the prediction of spectroscopic signatures of materials with fast turnaround.
Numerical simulations of the physical processes that occur in materials when they are excited with light are essential for the understanding and rational design of broad classes of materials for energy, quantum computation, and sensing applications. However, computationally intensive workflows are currently limiting the applicability of first-principles methods to complex systems, effectively hindering real-time identification and interpretation of experimentally observed physical processes. This project seeks to address this challenge by developing an AI-informed computational framework that integrates machine learning into existing workflows, thereby enabling the prediction of spectroscopic signatures of materials with fast turnaround.