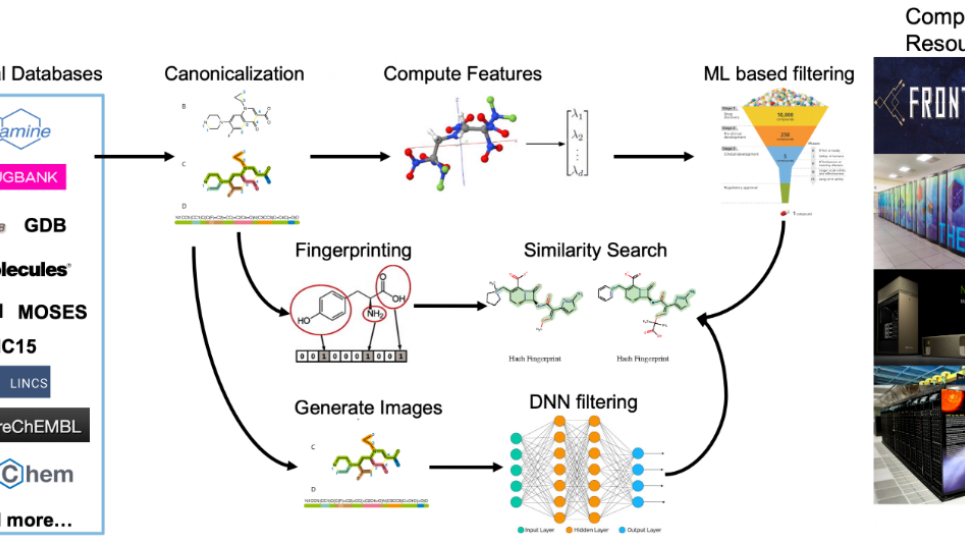
This project consists of developing and implementing a novel in silico drug design method coupling ML and physics-based methods.
Since the outbreak of COVID-19, researchers around the globe have been attempting to develop drugs that target specific the viral protein that are vital for its propagation. However, the drug discovery process employed in the pharmaceutical industry typically requires about 10 years and $2-3 billion for a single new drug, which is obviously not useful in emergencies like this pandemic. Machine learning (ML) techniques are increasingly being used to overcome this bottleneck. Recent developments in deep learning (DL) allow generation of novel drug-like molecules in silico by extensive sampling of the chemical space of relevance. However, their reliability depends heavily on the training data available; when insufficient reduces their effectiveness. Fortunately, physics-based and ML methods are complementary and, hence, combining these two should provide a very efficient way to predict binding affinities.
Coveney's team will develop and implement a novel in silico drug design method coupling ML and physics-based methods. It is important to note that our workflow is already set up and functional on Summit. Candidates will be sampled from both a billion-compound synthetically accessible space (including Enamine REAL) and selected from the output of a DL generative algorithm. The selected compounds will be scored using physics-based methods based on the binding free energies calculated and this information then be fed back to the DL algorithm for active learning, thereby refining its predictive capability. This loop will proceed iteratively involving a variety of physics-based scoring methods with increasing level of accuracies at each step ensuring that the DL algorithm gets progressively more accurate in its predictions. Augmenting human intelligence with artificial intelligence (AI) by supplementing chemists' knowledge can substantially reduce the throughput time for exploring this huge chemical space and hence improve the efficacy of exploration of real and virtual chemical libraries. The ongoing COVID-19 crisis has exposed severe limitations in the current pharmaceutical mode of drug discovery and it is imperative to overturn it to urgently develop a drug. This project is designed to accelerate the required transformation.