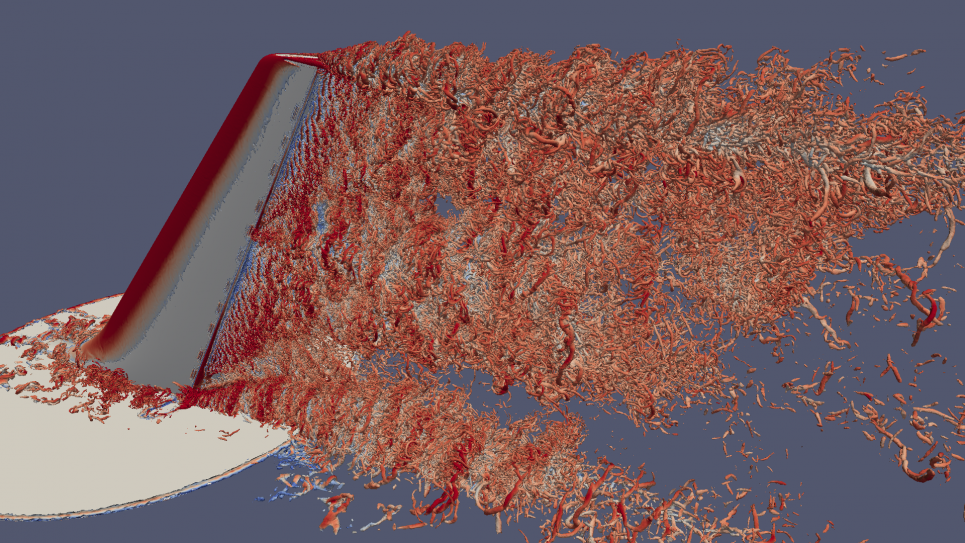
Flow over a vertical tail/rudder assembly (grey surface) with 24 active synthetic jets which introduce vortical structures (visualized by isosurfaces of vorticity colored by local flow speed) that alter the flow, reducing separation, improving rudder performance, and thereby allowing future aircraft designs to lower drag and fuel consumption. Image: Jun Fang, Argonne National Laboratory