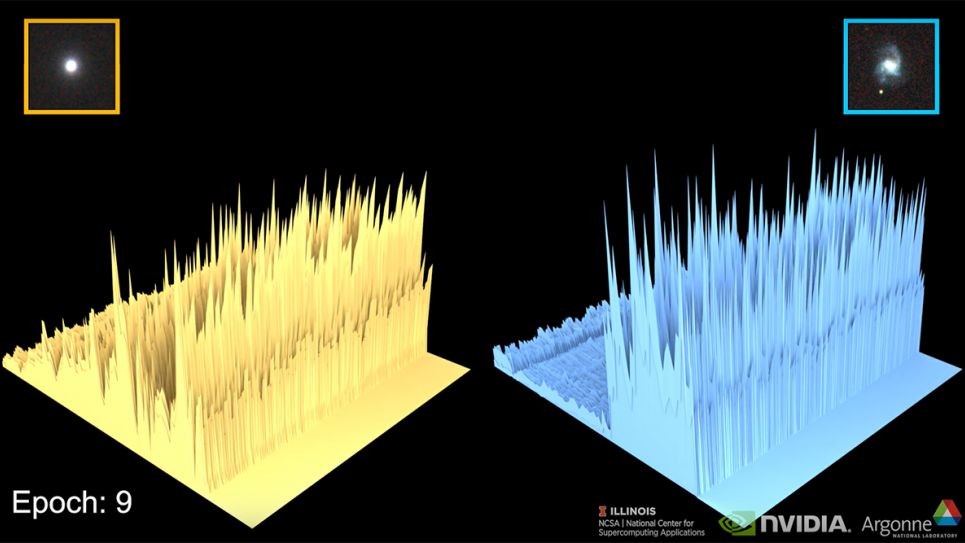
This image is a snapshot from a visualization that shows the output of the penultimate layer of a deep neural network during training as it learns to classify spiral and elliptical galaxies. Credit: Janet Knowles, Joseph A. Insley, and Silvio Rizzi, Argonne National Laboratory