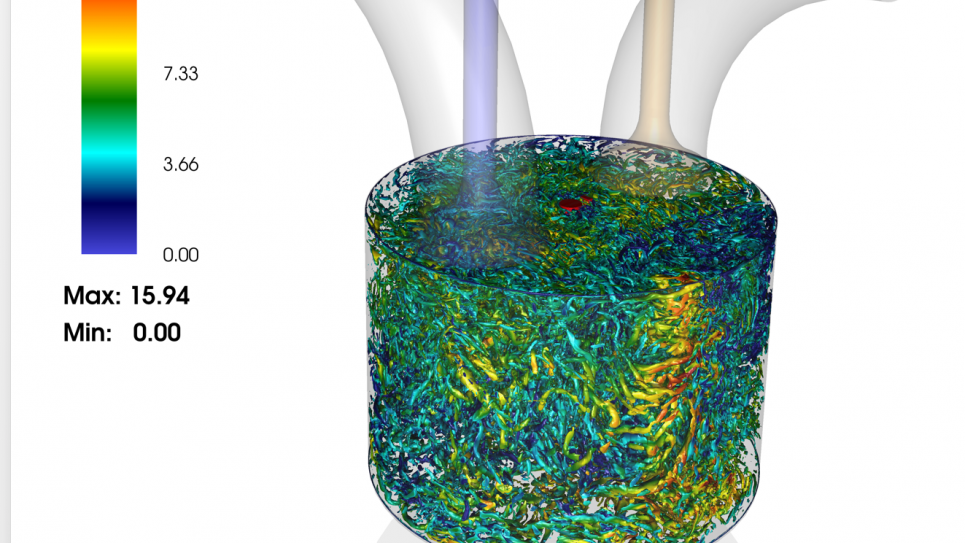
This project establishes a progressive hierarchy of detailed simulation campaigns of internal combustion engines that will enable scientific discovery and development of predictive models for cycle-to-cycle variability.
A key scientific challenge surrounding internal combustion engines (ICE) is the understanding, modeling, and control of cycle-to-cycle variability (CCV) in engine performance. CCV contributes to unevenness in the running of the engine, excessive engine noise and emissions, and engine knock, which can be potentially damaging. This project aims to develop a detailed understanding of the origins of CCV in a laboratory-scale modern engine using a combination of large eddy simulation (LES) and direct numerical simulation (DNS) techniques. While the high-fidelity LES will provide “gold-standard” data to understand root causes for CCV, the DNS will provide “ground truth” data for portions of the engine cycles to improve heat-transfer and combustion models further.
Establishing a progressive hierarchy of detailed simulation campaigns that will enable scientific discovery and development of predictive models, this project involves five main tasks: (1) Performing multi-cycle wall-resolved LES of a Direct-Injection Spark-Ignition (DISI) engine at motored conditions. (2) DNS of the compression strokes of a few select engine cycles to fully resolve the flow-field to assess the tumble breakdown process in more detail than have ever been captured before. (3) Wall-resolved LES with fuel injection to study the mechanism of momentum transfer between the fuel jet and the large scale in-cylinder tumble and swirl structures. (4) and (5) Multi-cycle, wall-resolved LES of the DISI engine at fired operating conditions for well-mixed and partially stratified operating conditions. The “gold-standard” LES and “ground truth” DNS datasets will be archived so that other researchers can access the data.The knowledge gained from this project will improve our understanding of ICE and help in developing more efficient and cleaner engines.