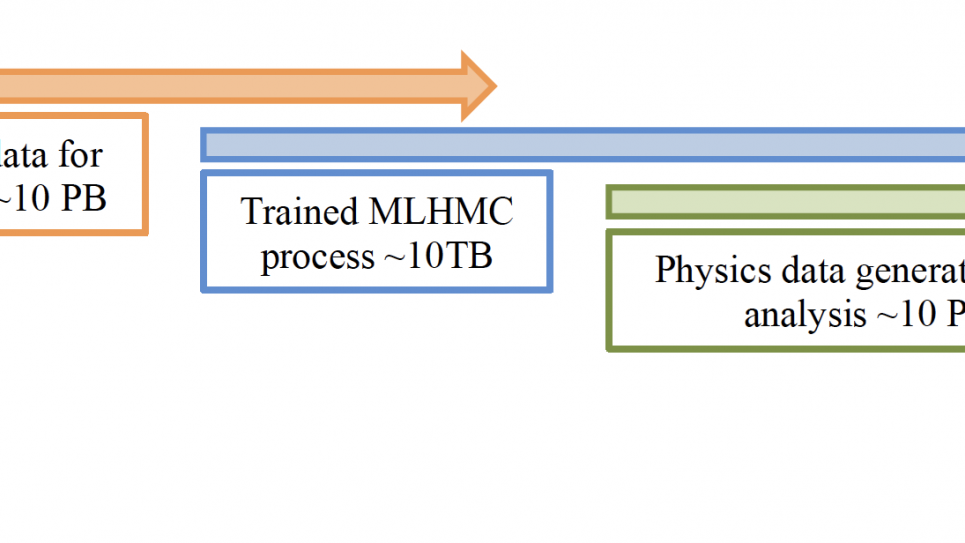
This project will determine possible interactions between nuclei and a large class of dark matter candidate particles. By coupling advanced machine learning and state-of-the-art physics simulations, it will provide critical input for experimental searches aiming to unravel the mysteries of dark matter while simultaneously giving insight into fundamental particle physics.