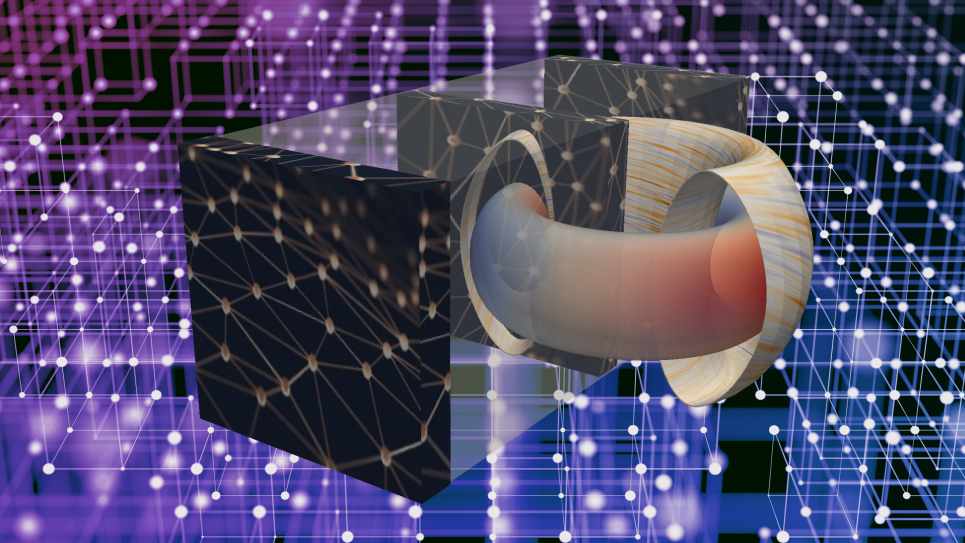
The team’s Fusion Recurrent Neural Network uses convolutional and recurrent neural network components to integrate both spatial and temporal information for predicting disruptions in tokamak plasmas. Image: Julian Kates-Harbeck, Harvard University; Eliot Feibush, Princeton Plasma Physics Laboratory