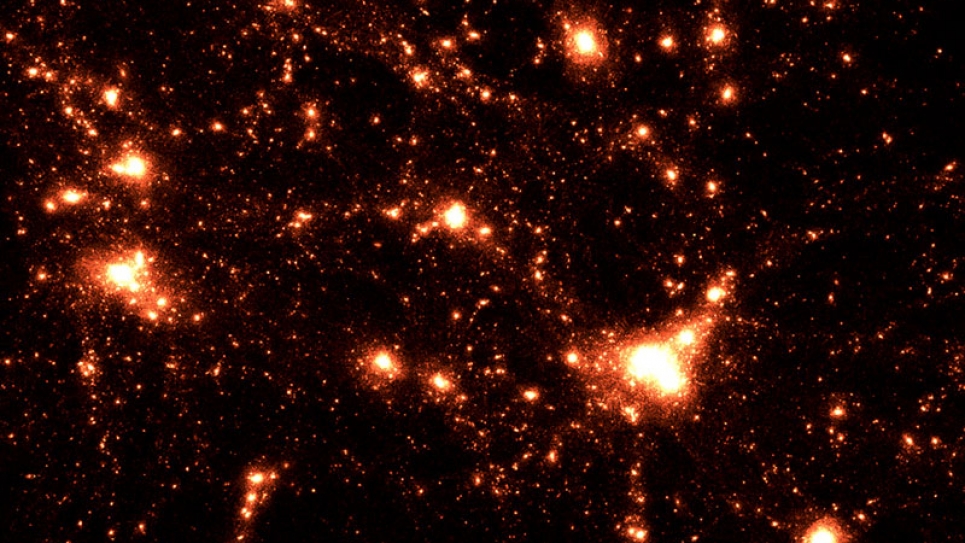
HACC cosmology simulations allow researchers to track the evolution of structures in great detail. Image: Joseph A. Insley, Silvio Rizzi, and the HACC team, Argonne National Laboratory
This project will connect some of the world’s largest and most detailed extreme-scale cosmological simulations with large-scale data obtained from the Legacy Survey of Space and Time (LSST) conducted at the Rubin Observatory. This astronomical survey will provide some of the most comprehensive observations of the visible sky to date. The simulations complement the observations by enabling unique probes of the universe using computations based on the underlying physics. By implementing cutting-edge data-intensive and machine learning techniques, this combined approach will usher in a new era of cosmological inference targeted at scientific breakthroughs.
Modern cosmology provides a unique window to fundamental physics, and has led to remarkable discoveries culminating in a highly successful model for the dynamics of the Universe. The three cosmological pillars—dark energy, dark matter, and inflation—are themselves signposts to further breakthroughs, as all reach beyond currently established theoretical boundaries. A new generation of sky surveys will soon shed light on these fundamental mysteries. In order to reach their full power, however, these observations must be combined with exascale simulations of cosmic structure formation. This project will exploit the unique architecture of the Aurora system, which will provide the platform for this synthesis, by constructing an ecology of data-intensive applications powered by cutting-edge statistical and machine learning techniques. This work will connect the extreme-scale simulations delivered by the ExaSky team within the Exascale Computing Project to large-scale data from the Legacy Survey of Space and Time (LSST), thereby enabling a new generation of scientific breakthroughs.
Next-generation cosmological surveys pose an extreme challenge to our modeling and prediction capabilities on small cosmological scales. At these scales, careful and precise modeling of nonlinear structure formation and the connection between the dark matter and the galaxy distribution in the Universe is essential to enable the extraction of the full cosmological information available in these rich and statistically exquisite datasets. By using forward modeling techniques enabled by modern machine learning approaches, as applied to exascale simulation data sets, this project will focus on using Aurora as the key resource to meeting this challenge.