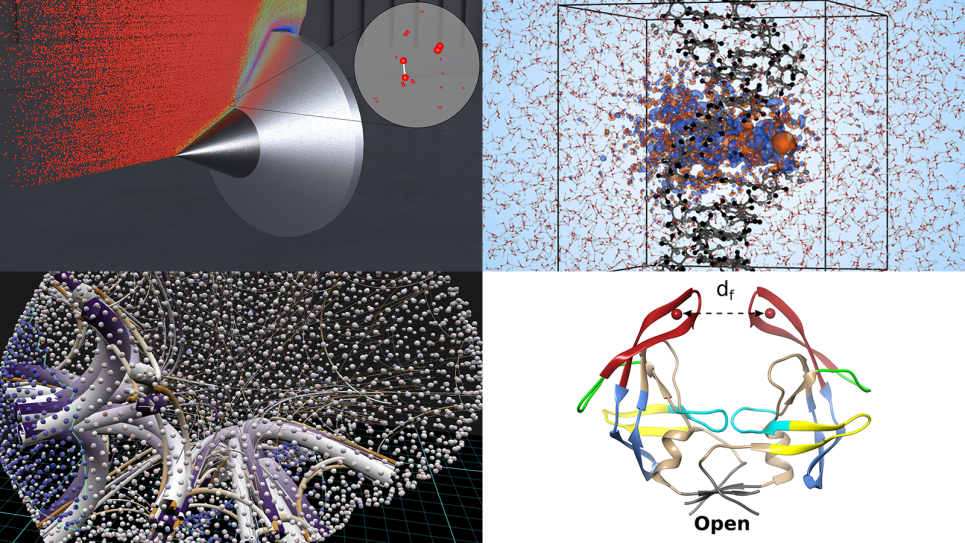
Over the past year, the ALCF user community produced a number of groundbreaking studies in areas ranging from hypersonic flight to climate science.
In 2023, researchers from across the globe leveraged supercomputing resources at the U.S. Department of Energy’s (DOE) Argonne National Laboratory to accelerate discoveries and innovation across a range of science areas.
From shedding light on protein dynamics to using artificial intelligence (AI) to speed up materials discovery, research projects supported by the Argonne Leadership Computing Facility (ALCF), a DOE Office of Science user facility, produced a number of impactful publications and studies.
The ALCF also made considerable progress in standing up the Aurora exascale supercomputer, completing the hardware installation and registering early performance numbers at the SC23 conference via a partial system run. In addition, the facility continued to expand the ALCF AI Testbed, rolling out new and upgraded AI systems for the research community. And with the launch of Argonne’s Nexus effort, the ALCF team also made strides toward the development of an integrated research infrastructure that connects supercomputers with experiments to streamline data-intensive science.
As 2024 approaches, we take a look back at some of the noteworthy research campaigns carried out by the ALCF user community over the past year. For more examples of the scientific advances enabled by ALCF computing resources, check out the 2023 ALCF Science Report.
Identifying reaction coordinates for key protein mechanism
Researchers from the University of Illinois Chicago used the ALCF’s Theta supercomputer to identify the exact reaction coordinates for a complex protein for the first time. Their work focused on the flap opening of HIV-1 protease — an essential process of this key viral protein and a major drug target. The team’s study marks an important step toward understanding protein functional dynamics. Their findings have far-reaching implications for both biomedical research and protein engineering, providing insights that are crucial for designing drugs, fighting drug resistance, and developing artificial enzymes that can carry out desired functions.
Accelerating the search for new catalysts
An Argonne team developed a new AI-driven model to accelerate the search for efficient and economically viable catalysts. Using simulations carried out on Theta, the team created a database of 20,000 structures for the binding energies of oxygen to doped molybdenum carbide. The database was then used to train a deep learning model for fast and accurate predictions of oxygen binding energies as the descriptors for catalytic stability. Instead of being limited to evaluating a few thousand catalyst structures over months with conventional computational methods, the team’s deep learning model can be used for precise and inexpensive calculations for tens of thousands of structures in milliseconds.
Enabling the discovery of new battery electrolyte
Researchers from Argonne performed simulations on Theta to aid in the discovery of a new fluoride-containing battery electrolyte that could protect next-generation batteries against performance decline. The team used simulations and experiments to better understand the behavior of the fluoride electrolyte at the atomic scale. Their findings allowed them to create a solid-electrolyte-interphase layer with optimal properties that maintains strong performance for hundreds of charge-discharge cycles. The new electrolyte offers many advantages for electric vehicles including high performance, stability, low cost, and safety.
Simulating hypersonic flows
To advance hypersonic flight research, a team from the University of Dayton Research Institute and Air Force Research Laboratory is using ALCF supercomputers to shed light on the complex thermal environments encountered by hypersonic vehicles. This year, the researchers published two studies detailing the molecular-level behavior of gas flows over shapes of relevance to hypersonic flight. Their work included performing large-scale simulations of oxygen flowing over a double cone and nitrogen flowing over a blunt wedge. The team’s research is helping to advance our understanding of the complex aerothermodynamics of hypersonic flight, providing insights that could help inform the design of safer and efficient technologies for national security, aviation, and space exploration.
Advancing climate modeling research
An Argonne team leveraged ALCF computing resources for multiple studies that provide a deeper understanding of changing climate conditions. In collaboration with ComEd, the researchers used dynamically downscaled climate models to produce detailed projections that are helping the company understand the potential impacts of climate change on its distribution grid and develop adaptation strategies. In another effort, the team performed six decade-long simulations to create a first-of-its-kind dataset that can be used to assess future flooding risks resulting from climate change.
Employing machine learning to predict materials properties
Researchers from Argonne and Oak Ridge national laboratories continue to use DOE supercomputers to advance the capabilities of the QMCPACK code for understanding and predicting the behavior and properties of a wide range of molecules and materials. Leveraging the ALCF’s Theta supercomputer, the team demonstrated how coupling QMCPACK with quantum machine learning methods can be used to predict the energetics of large molecules at a reduced computational cost while maintaining chemical accuracy. The team’s new machine learning-driven framework will enable researchers to study larger systems and predict the properties of molecules and materials more accurately, which could lead to advances in fields such as materials science, drug discovery, and energy research.
Understanding the impact of radiation on DNA
A team from the University of North Carolina is carrying out simulations on ALCF supercomputers to understand how DNA reacts when exposed to high-energy protons, like those used in ion-beam cancer treatments. Using Theta, the team explored the quantum-mechanical details of the energy transfer from high-energy protons to DNA in water. They found that the protons transfer more energy onto the DNA sugar-phosphate side chains than onto the nucleobases, resulting in damage to the DNA’s structure. Ultimately, the team’s research into the electronic excitation response of DNA aims to help scientists improve cancer therapies that use high-energy protons.
Modeling next-generation nuclear reactors designs
With help from ALCF supercomputers, Argonne researchers are working to advance the development of a next-generation nuclear power plant based on lead-cooled fast reactor technology. The team is using Theta to provide insights into the reactor’s flow physics and heat transfer mechanism. In a new study, the researchers showed that the selection of the appropriate Prandtl number (the ratio of momentum diffusivity to thermal diffusivity) significantly impacts the accuracy of simulations for advanced nuclear reactors. In addition, the team’s research provides valuable high-fidelity reference data that can be used by the nuclear reactor research community to validate and calibrate less computationally expensive models.
Improving atomistic simulations of materials
Scientists from the University of Southern California developed a new AI-driven method for simulating the behavior of atoms in materials more accurately and more quickly than traditional approaches. The method, called Allegro-Legato, employs machine learning to provide drastically improved fidelity scaling, which enables researchers to carry out larger and longer-duration quantum molecular dynamics simulations. The team used the ALCF’s Polaris supercomputer to demonstrate the capabilities and scalability of the new approach by simulating the vibrational properties of ammonia — a promising chemical for green fuel technologies.
Producing award-winning research papers
ALCF staff members contributed to multiple papers that garnered awards at computing conferences this year. At the 2023 IWOCL & SYCLcon conference, an ALCF team received Most Outstanding Paper for their work to standardize complex numbers in SYCL. Another ALCF team won first place and “best workflow” at the IEEE 2023 SciVis Contest for their development of a multi-platform scientific visualization application for analyzing data from brain plasticity simulations. At the ISAV workshop at SC23, ALCF researchers were recognized with the Best Paper Award for their efforts to instrument the NekRS computational fluid dynamics code with in situ visualization and analysis capabilities. Also at SC23, ALCF staff members were part of a team that received the Best Paper Award at the Workshop on Extreme-Scale Experiment-in-the-Loop Computing (XLOOP) for a study that demonstrated cross-facility data processing at scale. Finally, ALCF researchers contributed to a study on a new overhead-reducing approach to Bayesian optimization that won the Best Paper Award at the 2023 IEEE International Conference on eScience.